|
 |

|
Tutorials' Rooms
MORNING TUTORIALS 08.30-12.30
|
|
|
|
AFTERNOON TUTORIALS 14.00-18.00
|
|
|
|
|
|
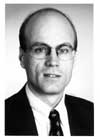 |
Björn Ottersten Email:
Royal Institute of Technology (KTH), Stockholm
Mats Bengtsson Email:
|
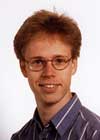
|
Multi-Antenna Communications
Abstract
Multiple antennas at the transmitter and/or receiver can be exploited in many different ways to improve the performance of wireless systems, in terms of reliability, throughput and coverage. This tutorial will cover various link and system-level issues of MIMO systems, ranging from channel characterization to interference handling.
Table of contents:
- MIMO Channel characterization and modeling. Channel rank, stochastic geometric and non-geometric models.
- The capacity of a MIMO link
- Some common misconceptions
- Basic transmission options, with and without channel knowledge at the transmitter.
- Exploiting partial channel information at the transmitter, using covariance feedback or noisy, quantized or outdated channel information.
- Interference handling at the receiver and transmitter.
- Space Division Multiple Access. Downlink beamforming, scheduling in time, space and frequency, opportunistic schemes.
Biography
Björn Ottersten received the M.S. degree in electrical engineering and applied physics from Linköping University, Linköping, Sweden, in 1986. In 1989 he received the Ph.D. degree in electrical engineering from Stanford University, Stanford, CA. Dr. Ottersten has held research positions at the Department of Electrical Engineering, Linköping University, the Information Systems Laboratory, Stanford University, and the Katholieke Universiteit Leuven, Leuven. During 96/97 Dr. Ottersten was Director of Research at ArrayComm Inc, San Jose, California. He has authored papers that received the Signal Processing Society Paper Award in 1993 and 2001. In 1991 he was appointed Professor of Signal Processing at the Royal Institute of Technology (KTH), Stockholm and he is currently dean of the School of Electrical Engineering at KTH. From 1992 to 2004 he was head of the department for Signals, Sensors, and Systems at KTH. Dr. Ottersten is also a visiting professor at the University of Luxembourg. Dr. Ottersten has served as Associate Editor for the IEEE Transactions on Signal Processing and a member of the editorial board of EURASIP Journal of Applied Signal Processing. He is currently editor in chief of EURASIP Signal Processing Journal and a member of the editorial board of IEEE Signal Processing Magazine. Dr. Ottersten is a Fellow of the IEEE. His research interests include wireless communications, stochastic signal processing, sensor array processing, and time series analysis.
Mats Bengtsson received the M.S. degree in computer science from Linköping University in 1991 and the Tech. Lic. and Ph.D. degrees in electrical engineering from the Royal Institute of Technology (KTH), Stockholm, Sweden, in 1997 and 2000, respectively. From 1991 to 1995, he was with Ericsson Telecom AB Karlstad. He currently holds a position as Research Associate at the Royal Institute of Technology. His research interests include statistical signal processing and its applications to antenna array processing and communications, radio resource management and propagation channel modelling.
|
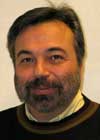 |
Prof. Georgios B. Giannakis
Dept. of ECE, Univ. of Minnesota Minneapolis, MN 55455; USA
Email:
|
Ultra Wideband Communications: From Concept to Reality
Abstract
In February 2002, a law-and-order of the Federal Communications Commission (FCC) gave the "green light'' (spectral mask in therange 3.1-10.6 GHz) for commercial applications of Ultra Wideband (UWB) systems. Since this recent FCC release, UWB has emerged asan exciting technology whose "time has come'' for wireless communications, and local area networking. Companies (such as TimeDomain, AetherWire, and XtremeSpectrum) have recently produced chipsets for UWB communication systems. UWB technology, referring to bandwidth exceeding 2GHz or fractional bandwidth of more than25\, is attracting increasing interest in academia, industry and government labs. Conveying information over Impulse-like Radio (IR) waveforms, UWB technology comes with unique features: low-power carrier-free transmissions, ample multipath diversity, low-complexity baseband transceivers and a potential for increase in capacity. The scarcity of bandwidth resources coupled with the capability of IR to overlay existing systems, welcomes UWB connectivity in the workplace, and at home for indoor and especially short range wireless links. Utilizing ultra-short pulses, UWB also allows for very accurate delay estimates providing position and location capabilities within a few centimeters. However, to realize these attractive features, UWB research and development has to cope with formidable challenges that include: high sensitivity to timing the reception of ultra-short pulses, mitigation of fading propagation effects with pronounced frequency-selectivity, low-complexity constraints in decoding high-performance multiple access protocols, and strict power limitations imposed by the desire to minimize interference between UWB communicators, and co-existing RF systems. These challenges call for advanced signal processing expertise in UWB communications - a view also shared by government agencies andindustry. Testament to this growing trend towards signal processing topics for UWB related applications is also provided by the number of UWB sessions in conferences, and plenary talks devoted to this subject.Responding to such an interest, this tutorial will provide a comprehensive overview of the state-of-the-art in UWB communications with emphasis on the unique features, challenges, and research directions tailored to signal processing aspects.
The contents of the proposed tutorial will be structured as follows:
I. Introduction; II. History of UWB; III. Motivating Applications; IV. UWB Communications at the Physical Layer;
IV-A Transmitter Design; IV-B Synchronization; IV-C Channel Modeling and Estimation; IV-D Receiver Design; IV-E Multiple Access and Interference Suppression;
V. UWB Communications at the Networking Layer; VI. Implementation Issues; VII. Conclusions and Open Problems; VIII.Extensive Bibliography.
Intended Audience
Graduate students, researchers and engineers with general interests in Communications, Signal Processing, Information Theory, and specific interests in ultra-wideband diversity techniques, fading countermeasures, space-time processing, mobile, multiple access, as well as UWB implementation, and networking aspects. The background needed is that of an M.Sc. Degree holder or commensurate experience with random processes, linear algebra, statistical signal processing, detection-estimation, and basic information theory and coding concepts.
Biography
GEORGIOS B. GIANNAKIS (Fellow'97) G. B. Giannakis received his Diploma in Electrical Engineering from the National Technical University of Athens, Greece, 1981. September 1982 to July 1986 he was with the University of Southern California (USC), where he received his MSc. in Electrical Engineering, 1983, MSc. in Mathematics, 1986, and Ph.D. in Electrical Engineering, 1986. After lecturing for one year at USC, he joined the University of Virginia in 1987, where he became a professor of Electrical Engineering in 1997. Since 1999 he has been a professor with the Department of Electrical and Computer Engineering at the University of Minnesota, where he now holds an ADC Chair in Wireless Telecommunications. His general interests span the areas of communications and signal processing, estimation and detection theory, time-series analysis, and system identification -- subjects on which he has published more than 250 journal papers, 400 conference papers and two edited books. Current research focuses on diversity techniques for fading channels, complex-field and space-time coding, multicarrier, ultra-wide band wireless communication systems, cross-layer designs and sensor networks. G. B. Giannakis is the (co-) recipient of six paper awards from the IEEE Signal Processing (SP) and Communications Societies (1992, 1998, 2000, 2001, 2003, 2004). He also received Technical Achievement Awards from the SP Society in 2000 and from EURASIP in 2005. He served as Editor in Chief for the IEEE SP Letters, as Associate Editor for the IEEE Trans. on Signal Proc. and the IEEE SP Letters, as secretary of the SP Conference Board, as member of the SP Publications Board, as member and vice-chair of the Statistical Signal and Array Processing Technical Committee, as chair of the SP for Communications Technical Committee and as a member of the IEEE Fellows Election Committee. He has also served as a member of the IEEE-SP Society's Board of Governors, the Editorial Board for the Proceedings of the IEEE and the steering committee of the IEEE Trans. on Wireless Communications.
|
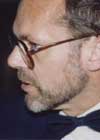 |
Ezio Biglieri Departament de Tecnologia Universitat Pompeu Fabra
Email: , |
Iterative ('turbo') algorithms on factor graphs: Theory and applications
Abstract
Originally developed for decoding turbo codes, normal factor graphs are a natural setting for the description of iterative techniques for detecting coded signals transmitted on a variety of channels. In addition, they provide a unified framework allowing one to understand the connections among seemingly different detection problems. This tutorial describes the application of normal factor graphs to a number of these problems, such as equalization of coded signals, multiuser detection, decoding of multilevel coded modulation, and reception of space--time coded signals.
1. Soft Decoding. Factor graphs and the Sum--Product algorithm. 2. Decoding codes described by factor graphs. The turbo algorithm. 3. Low-density parity-check codes. Turbo codes. 4. Analysis of turbo algorithms: EXIT charts. 5. Turbo algorithms for multiuser detection. 6. Turbo algorithms for space--time decoding in MIMO systems. 7. Turbo algorithms for coded modulation.
Biography
EZIO BIGLIERI is presently a professor with Universitat Pompeu Fabra, Barcelona, Spain. His previous positions include professorships with Universita` di Napoli (1975--1977), with UCLA (1987--1989), and with Politecnico di Torino (1977--1987 and 1990--2005). He has held visiting positions with the Department of System Science, UCLA, the Mathematical Research Center, Bell Laboratories, Murray Hill, NJ, the Bell Laboratories, Holmdel, NJ, the Department of Electrical Engineering, UCLA, the Telecommunication Department of The Ecole Nationale Superieure des Telecommunications, Paris, France, the University of Sydney, Australia, the Yokohama National University, Japan, the Electrical Engineering Department of Princeton University, the University of South Australia, Adelaide, the University of Melbourne, the Institute for Communications Engineering, Munich Institute of Technology, Germany, and the Institute for Infocomm Research, National University of Singapore. He was elected three times to the Board of Governors of the IEEE Information Theory Society, and served as its President in 1999. He was the general co-chairman of the "IEEE 2000 International Symposium on Information Theory," Sorrento, Italy, June 2000, and the general co-chairman of ISITA 2004, Parma, Italy. He is a Fellow of the IEEE, and a Distinguished Lecturer for the IEEE Information Theory Society and the IEEE Communications Society. He was an Editor of the IEEE Transactions on Communications, the IEEE Transactions on Information Theory, the IEEE Communications Letters, the Journal on Communications and Networks, and the Editor in Chief of the European Transactions on Telecommunications. Since 2004 he has been the Editor-in-Chief of the IEEE Communications Letters. He has published 6 books and in excess of 120 journal papers on digital communications. Among other honors, he received the IEEE Third-Millennium Medal, the IEEE Donald G. Fink Prize Paper Award, and the IEEE Communications Society Edwin Howard Armstrong Achievement Award.
|
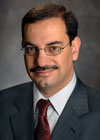 |
Ali H. Sayed
Electrical Engineering Department UCLA
Email:
|
Energy Conservation in Adaptive Filtering
Abstract
Adaptive filters are systems that respond to variations in their environment by adapting their internal structure in order to meet desired performance specifications. Adaptive filters are endowed with both learning and tracking abilities that make them extremely useful in a variety of applications ranging from signal processing, to communications, biomedical engineering, consumer products, and military electronics. This tutorial discusses three emerging trends in the study of adaptive filters in view of the increased degrees of mobility and complexity in modern applications. One trend relates to a shift in emphasis from the design of adaptive filters to the design of interactive adaptive blocks within complex systems, especially within distributed networks. A second trend relates to the need to endow adaptive filters with more complex learning mechanisms that can extract and exploit information about the surrounding environment. And a third trend relates to the need to characterize more fully the limits of performance of existing schemes and to develop variants that can meet more stringent specifications. The performance of an adaptive filter is traditionally evaluated in terms of its transient behavior and its steady-state behavior. The former provides information about how fast a filter learns, while the latter provides information about how well a filter learns. Such performance analyses tend to be challenging since adaptive filters are, by design, time-variant, nonlinear, and stochastic systems. For this reason, it has been common in the literature to study different adaptive schemes separately and under different assumptions due to the variations that exist in their update equations. This tutorial provides an overview of an energy conservation approach to the performance analysis of adaptive filters. The framework is based on studying the energy flow through successive iterations of an adaptive filter and on establishing a fundamental energy conservation relation; the relation bears resemblance with Snell?s Law in optics and has far reaching consequences on the study of adaptive schemes. In this way, many new and old results can be pursued uniformly across different classes of algorithms. The analysis will also highlight some interesting phenomena regarding the learning ability of adaptive filters. It will be seen that adaptive filters generally learn at a rate that is better than that predicted by least-mean-squares theory; that is, they are "smarter" than originally thought! It will also be seen that adaptive filters actually have two distinct rates of convergence; they learn at a slower rate initially and at a faster rate later; perhaps in a manner that mimics the human learning process. The tutorial will also provide an overview of recent work on adaptive distributed systems that are able to exploit the temporal and spatial dimensions of the data collected at spatially distributed nodes. Such distributed networks will form the backbone of future data communication and control networks. Applications will range from sensor networks to precision agriculture, environment monitoring, disaster relief management, smart spaces, and medical applications. In all these cases, the distribution of the nodes in the field yields spatial diversity, which should be exploited by the adaptive filters alongside the temporal dimension in order to enhance the robustness of the processing tasks and improve the probability of signal and event detection. Adaptation is needed not only because the environmental conditions vary with time and space, but also because the network topology may vary. Adaptive distributed structures will be described and their performance will be examined by means of energy conservation arguments.
Biography
Ali H. Sayed received his PhD in Electrical Engineering from Stanford University in 1992. He is Professor and Chairman of Electrical Engineering at UCLA where he also directs the Adaptive Systems Laboratory. He has published widely in the areas of adaptive filtering, estimation theory, and signal processing for communications with over 250 articles and 4 books, including the textbook Fundamentals of Adaptive Filtering (Wiley, NY, 2003). He is a Fellow of IEEE and has served as Editor-in-Chief of the IEEE Transactions on Signal Processing (2003-2005). He serves as Editor-in-Chief of the EURASIP Journal on Applied Signal Processing. His research has received several recognitions including the 1996 IEEE Donald G. Fink Prize, 2002 Best Paper Award from the IEEE Signal Processing Society, 2003 Kuwait Prize, 2005 Frederick E. Terman Award, and two Best Student Paper Awards at international meetings (1999,2001). He has served as a 2005 Distinguished Lecturer of the IEEE Signal Processing Society and as a member of the Publications (2003-2005) and Award (2005) Boards of the same society. He is a member of the Signal Processing Theory and Methods (SPTM) and Signal Processing for Communications (SPCOM) technical committees of the IEEE Signal Processing Society. He is also serving as General Chairman of ICASSP 2008 to be held in Las Vegas.
|
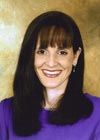 |
Yonina C. Eldar
Department of Electrical Engineering Technion, Israel Institute of Technology Haifa, Israel
Email:
|
Numerical Optimization Methods for Robust Parameter Estimation
Abstract
In recent years there has been a surge of research in robust optimization and interior point methods within the mathematical programming community, leading to new powerful optimization techniques as well as efficient software packages. These advances have begun impacting various applied fields, where computational efficiency and the ability to deal with uncertainties is of paramount importance. The goal of this tutorial is to introduce new methods for robust parameter estimation by applying these modern optimization tools to solving core problems in statistical estimation theory.
The first part of the tutorial will focus on the problem of estimating an unknown deterministic parameter vector in a linear model. Instead of utilizing data error methods, which can often lead to a large estimation error, we discuss a mean-squared error (MSE) framework for designing robust estimators with good MSE behavior. As we demonstrate, this framework leads to new, powerful estimation methods that can significantly outperform existing estimators such as least-squares and Tikhonov regularization. We also treat the problem of evaluating the performance of different estimators based on the concepts of admissible and dominating estimators. We then show how these ideas can be extended to more general, nonlinear models by developing bounds on the MSE that are smaller than the traditional Cramer-Rao lower bound for all values of the unknowns. The method of development we present inherently leads to explicit constructions of estimators that achieve these bounds in cases where an efficient estimator exists, by performing a simple linear transformation on the standard maximum likelihood (ML) estimator. This leads to estimators that result in a smaller MSE than the ML method for all possible values of the unknowns.
In the second part of the tutorial we focus on Bayesian estimation of random parameters in the presence of model uncertainties. We describe two approaches for estimation in this context: minimax MSE and minimax regret. The latter is a competitive approach, which seeks the estimator whose performance in terms of MSE is as close as possible to that of the optimal estimator for the case in which the model is known exactly. The advantage of this strategy is that it is often less conservative than the conventional minimax MSE approach, and can account for more general uncertainty regions.
Outline:
Introduction:
Estimation Models Background on convex analysis
Deterministic Parameter Estimation:
Linear estimation in linear models
Data error approaches MSE-based methods
Minimax and blind minimax MSE estimation Minimax regret estimation Admissible and dominating estimation methods Maximum set estimators Estimation in nonlinear problems
Uniform CRLB Improving the CRLB and maximum-likelihood estimation
Random Parameter Estimation:
Finite-dimensional model with model uncertainties Minimax regret filtering
Biography
Yonina C. Eldar received the B.Sc. degree in Physics in 1995 and the B.Sc. degree in Electrical Engineering in 1996 both from Tel-Aviv University (TAU), Tel-Aviv, Israel, and the Ph.D. degree in Electrical Engineering and Computer Science in 2001 from the Massachusetts Institute of Technology (MIT), Cambridge.
From January 2002 to July 2002 she was a Postdoctoral Fellow at the Digital Signal Processing Group at MIT. She is currently an Associate Professor in the Department of Electrical Engineering at the Technion - Israel Institute of Technology, Haifa, Israel. She is also a Research Affiliate with the Research Laboratory of Electronics at MIT.
From 1992 through 1996 she was in the program for outstanding students in TAU. In 1998 she held the Rosenblith Fellowship for study in Electrical Engineering at MIT, and in 2000 she held an IBM Research Fellowship. She is currently a Horev Fellow in the Leaders in Science and Technology program at the Technion, and an Alon Fellow. In 2004, she was awarded the Wolf Foundation Krill Prize for Excellence in Scientific Research, and in 2005 the Andre and Bella Meyer Lectureship. She is a member of the IEEE Signal Processing Theory and Methods technical committee and an Associate Editor for the IEEE Transactions on Signal Processing.
|
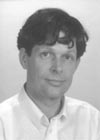 |
Hans-Andrea Loeliger
ETH Zurich, Switzerland
Email:
|
The Factor Graph Approach to Signal Processing
Abstract
Factor graphs (or similar graphical models) allow a unified approach to a number of topics in coding, signal processing, and machine learning. Many of the best algorithms in these fields may be viewed as message passing in a factor graph, and new efficient algorithms for detection/estimation problems in complex system models may be obtained by putting together tabulated message computation rules for the building blocks of the system model. The tutorial begins with an elementary introduction to factor graphs and the sumproduct and max-product algorithms for discrete variables. However, the focus of the tutorial is on algorithms for models with continuous variables. Special attention is given to multivariable Gaussian message passing on linear models, which includes Kalman filtering and smoothing, linear minimum mean squared error (LMMSE) estimation, recursive least squares (RLS), linear predictive coding (LPC) analysis, and related topics. Beyond the Gaussian case, the tutorial develops also the message passing approach to gradient methods, particle filters (sequential Monte Carlo methods), and expectation maximization. With its emphasis on ?local? computations, the factor graph approach encourages, and helps, to mix and match all these techniques.
Biography
Hans-Andrea Loeliger received a diploma in electrical engineering in 1985 and a Ph.D. in 1992, both from ETH Zurich, Switzerland. From 1992 to 1995 he was with Link¨oping University, Sweden. From 1995 to 2000, he was with Endora Tech AG, Basel, Switzerland, of which he is a cofounder. Since 2000, he has been a Professor at ETH Zurich. His research interests lie in the broad areas of signal processing, information theory, communications, and electronics. He is a fellow of the IEEE.
|
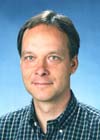 |
Michael Unser
Institute of Imaging and Applied Optics EPFL, Lausanne, Switzerland
Email:
|
The colored revolution of bio-imaging: new opportunities for signal processing
Abstract
During the past decade, biological imaging has undergone a revolution thanks to the development of highly specific fluorescent probes in conjunction with new high-resolution microscopes. Fluorescence microscopy is becoming widespread and is having a profound impact on the way research is being conducted in the life sciences. Biologists can now visualize sub-cellular components and processes in vivo, both structurally and functionally. Observations can be made in two or three dimensions, at different wavelengths (spectroscopy), possibly with time-lapse imaging to investigate cellular dynamics. Signal processing is at the heart of these developments and is expected to play an ever-increasing role in the field.
The goal of this tutorial is to introduce engineers to modern fluorescence microscopy while making them aware of corresponding research opportunities. The first part will cover the principles of fluorescence imaging, while the second will concentrate on signal processing aspects and challenges.
Part 1: Basics of Fluorescence Imaging
- Fluorescence labeling: the green/colored revolution - Image formation: widefield and confocal microscopy; PSF - Detectors and limiting factors
Part 2: Signal Processing Challenges
- Image preparation: calibration, feature detection - Restoration: denoising, 3D deconvolution, inverse problems - Registration and segmentation - Quantitative image analysis: motion; particle tracking; model fitting; classification
Biography
Michael Unser received the M.S. (summa cum laude) and Ph.D. degrees in Electrical Engineering in 1981 and 1984, respectively, from the Ecole Polytechnique Fédérale de Lausanne (EPFL), Switzerland. From 1985 to 1997, he worked as a scientist with the National Institutes of Health, Bethesda USA. He is now professor and Director of the Biomedical Imaging Group at the EPFL. His main research area is biomedical image processing. He has a strong interest in sampling theories, multiresolution algorithms, wavelets, the use of splines for image processing and is the author of over 120 published journal papers in these areas.
Dr. Unser is the associate Editor-in-Chief of the IEEE Transactions on Medical Imaging and the Editor-in-Chief of the Wavelet Digest, the electronic newsletter of the wavelet community. He was general chair for the first IEEE International Symposium on Biomedical Imaging (ISBI'2002), which was held in Washington, DC, July 7-10, 2002. He also chairs the newly created technical committee of the IEEE-SP Society on Bio Imaging and Signal Processing (BISP).
Dr. Unser is a fellow of the IEEE. He received the 1995 and 2003 Best Paper Awards and the 2000 Magazine Award from the IEEE Signal Processing Society.
|
|
 |
|
 |
|